Report for Sydney Water Corporation
Peer Review of Sydney Water's Demand
Forecast
24 June 2019
About the Author
is a Sapere director based in the Sydney. He is an experienced economist
and management consultant with expertise in economic regulation and policy, quantitative
analysis (including applied econometric analysis) and business management issues including
strategy, procurement and risk management. He has worked across a range of industries
including water, energy, transport, natural resources, insurance and other financial services.
About Sapere Research Group Limited
Sapere Research Group is one of the largest expert consulting firms in Australasia and a
leader in provision of independent economic, forensic accounting and public policy services.
Sapere provides independent expert testimony, strategic advisory services, data analytics and
other advice to Australasia’s private sector corporate clients, major law firms, government
agencies, and regulatory bodies.
Sydney
Canberra
Melbourne
Level 18, 135 King
GPO Box 252, Canberra Level 8, 90 Collins Street
Street, Sydney, NSW
City, ACT 2601
Melbourne VIC 3000
2000
, GPO Box 3179
Melbourne VIC 3001
Wellington
Auckland
Level 9, 1 Willeston St
Level 8, 203 Queen St
PO Box 587
PO Box 2475
Wellington 6140
Auckland 1140
For information on this report please contact:
Name:
Telephone:
Mobile:
Email:
Page i
Commercial in Confidence
Contents
Glossary ............................................................................................................... v
Executive summary ........................................................................................... vii
Introduction and overview . .................................................................................... vii
Residential forecast ................................................................................................... vii
Overview of the residential forecast ....................................................... vii
Assessment of the residential forecast .................................................... vii
Non-residential forecast . ........................................................................................ viii
Overview . .................................................................................................. viii
Assessment ................................................................................................. viii
1.
Introduction ............................................................................................ 1
2.
Background and context ......................................................................... 2
2.1
The need for forecasting requirements. ................................................... 2
2.1.1
Uses for demand forecasts ........................................................... 2
2.1.2
Requirements of the forecast ...................................................... 3
2.2
Sydney Water’s approaches to forecasting ............................................... 3
2.2.1
Residential segment ....................................................................... 3
2.2.2
Non-residential segment .............................................................. 4
3.
Review of the STDF for residential demand ........................................... 5
3.1
The overall approach to residential demand forecasting . ..................... 5
3.1.1
Description ..................................................................................... 5
3.1.2
Assessment of broad approach ................................................... 5
3.2
Econometric model for the residential demand forecast ...................... 6
3.2.1
Overview of the model ................................................................. 6
3.2.2
Assessment ..................................................................................... 8
3.3
Calibration and forecasting . ..................................................................... 13
3.3.1
Overview of the process ............................................................ 13
3.3.2
Assessment ................................................................................... 15
3.4
Aggregation of the forecast ...................................................................... 16
3.4.1
Overview....................................................................................... 16
3.4.2
Assessment ................................................................................... 16
3.5
Modelling results ........................................................................................ 16
3.6
Summary and conclusion .......................................................................... 18
4.
Review of the STDF for non-residential demand .................................. 19
4.1
The overall approach to non-residential demand forecasting ............ 19
4.1.1
Summary of approach ................................................................ 19
4.1.2
The 2015 forecast ........................................................................ 19
4.1.3
Current Forecast adjustments and modifications .................. 20
4.2
Details of the forecast ............................................................................... 21
4.2.1
Aspects of the 2015 forecast ..................................................... 21
Page iii
Commercial in Confidence
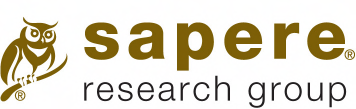
4.2.2
Running the forecast under a range of future weather
scenarios ........................................................................................ 23
4.2.3
Recent adjustments ..................................................................... 24
4.3
Assessment .................................................................................................. 24
4.3.1
The overall approach and implementation ............................. 24
4.3.2
The recent adjustments .............................................................. 27
4.3.3
Other billed consumption .......................................................... 30
4.3.4
Modelling results ......................................................................... 30
4.3.5
Conclusion .................................................................................... 31
Appendices
No table of contents entries found.
Tables
Table 1: Weather variables used and considered
8
Table 2: Price variables used
10
Table 3: Non-residential segments
21
Figures
Figure 1: Hindcast/forecast plus actual for all residential dwellings
17
Figure 2: Relative Hindcast error
18
Figure 3: Weather and forecast error
29
Figure 4: Summary of non-residential forecast
30
Page iv
Commercial in Confidence
Glossary
2013 Forecast
The non-residential forecast conducted in 2013 for the purposes of
supporting the LTDF
2014 Review
A review conducted by Sapere Research Group of the 2014 short
term demand forecast
2015 Forecast
A revised non-residential demand forecast used for the 2016
pricing determination period
CPI
Consumer price index
Current Forecast
The current non-residential demand forecast
DPE
Department of Planning and Environment
IPART
Independent Pricing and Regulatory Tribunal
LCD
Litres per capita per day
LTDF
Long term demand forecast
Methodology and
A paper titled ‘The Econometric Methodology and Results for
Results Paper
2018 Demand Forecasting Project for Sydney Water Corporation’,
13 November 2018 by Dr Vasilis Sarafidis (Monash University)
NARCliM Project
A research partnership between the NSW and ACT governments
and the Climate Change Research Centre at the University of
NSW, which produces future climate change projections
Sapere
Sapere Research Group
STDF
Short term demand forecast
Trends Paper
A short paper that examined the ‘Impact of adding trend values
and expanding estimation period’. Provided on 21 December 2018
by Dr Vasilis Sarafidis
Page v
Commercial in Confidence
Executive summary
Introduction and overview
Sydney Water Corporation (Sydney Water) needs to develop a short term demand forecast
(STDF) for urban water to support the Independent Pricing and Regulatory Tribunal
determination of usage and service charges for the four year period from 1 July 2020.
Sydney Water’s approach to the STDF involves separately forecasting non-residential,
residential demand and leakage. In this paper I provide a peer review of the residential and
non-residential components of the STDF with the objective of critically assessing the
adequacy and robustness of the models used to produce the forecast.
In my opinion—given the context and purpose—the forecasts are adequate and robust. I
have noted some concerns. However, I believe these to be minor, particularly relative to the
variability caused by weather.
Residential forecast
Overview of the residential forecast
The residential forecasting approach can be summarised as comprising three stages.
1.
Developing models of household water demand. Econometric models (for 34 different
property segments) were independently developed to model how household demand
varies with price, season and weather.
2.
Calibrating the models and forecasting average demand by household type. Sydney
Water calibrated the models and applied them to forecast average demand by household
type. The forecast assumes no change in the real usage price. The weather is estimated
using independently-developed weather-scenarios that account for climate change.
3.
Aggregating the forecasts using projections of dwelling numbers. Sydney Water
combined the forecast of average demand with forecasts of dwelling numbers (based on
data from the Department of Planning and Environment) to estimate an aggregate level
of demand by quarter to June 2025 by system.
The approach is very similar to that adopted for the STDF for the prior determination
period (July 2016 to June 2020). The most significant change is the use of additional weather
variables and independently-developed weather-scenarios as a basis for weather forecasts
(instead of using historical averages). As with the previous studies, the main contribution of
the models is in estimating the impact of season and weather on demand.
Assessment of the residential forecast
Given the available information, the approach adopted appears adequate and robust for the
purpose of the forecast.
Page vii
Commercial in Confidence
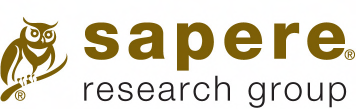
The greatest risk to forecast accuracy relates to the influence of weather. Sydney Water’s
modelling suggests that demand can vary by plus or minus 6 per cent during any one year
due to weather variation.
There is some evidence of a slight downward trend in average residential demand in some
segments that is not reflected in the modelling and the forecast. A possible explanation is
continual improvements in water efficiency. If such a trend (exists and) persists then the
forecast may slightly overestimate water demand. However, it is also possible there is no
persistent trend and the results are caused by some unexplained variation (e.g. weather
related not captured in the weather variables). Nevertheless, the effect is very small relative
to other sources of variation.
I also have a minor concern related to modelling the effect of price. The water usage price
only varied to a small extent over the estimation period and most variations in the ‘price’
variable were due to differences in measurements of the consumer price index (CPI).
Furthermore, the estimate of the impact of price was sensitive to the use of additional data.
Nevertheless, I consider any risks to be small. The estimates of the price impact appear
reasonable and I expect the modelling of price would have a small impact on the forecast.
Non-residential forecast
Overview
The non-residential forecast is essentially an extension of the previous forecast 2015
Forecast (which itself was a modification of the 2013 Forecast) with:
•
a different approach to forecasting weather; based on independently developed future
weather scenarios rather than historical average.
•
some adjustments to account for observed discrepancies between the previous forecast
and recent observed outcomes.
The 2013 Forecast—upon which the current forecast is based—involved:
•
forecasting average demand for 66 different property segment and system combinations
by estimating econometric models using historical data, and
applying the models to develop forecasts using assumptions on price and weather
•
forecasting property numbers based on an examination of trends
•
multiplying the above to estimate aggregate demand by segment and system.
The non-residential forecast is combined with ‘other’ billed meter consumption, which is
estimated based on historical averages.
Assessment
Sydney Water has re-used rather than re-estimated the 66 underlying models and property
forecasts developed in 2013. While this is not ideal, the accuracy of the forecast mostly
depends on the adjustments made and the weather forecast.
In my opinion the adjustments are not unreasonable. I have a slight concern that the models
used appear to underestimate the weather extremes. Due to this and another error, the
adjustment and the forecast may be slightly too large. The issues are, however, small.
Page viii
Commercial in Confidence
1. Introduction
Sydney Water Corporation (Sydney Water) needs to undertake forecasts of water demand to
support Independent Pricing and Regulatory Tribunal (IPART) determinations of usage and
service charges.
I have been engaged by Sydney Water to provide a peer review of the short term demand
forecasting (STDF) model. The purpose of the review is to ensure that these models used are
robust and accurate (fit-for-purpose).
This report provides the residential and non-residential demand components of the STDF.
The scope (see Box 1 below) is very similar to the review of the residential-demand
component conducted for Sydney Water STDF in 2014 and 2011. Given the similarity in
scope and the similarity of the models used, this review draws from the 2011 and 2014
Reviews.
The rest of the report is structured as follows:
•
The following section (Section 2) describes the background and context to the
forecasts.
•
Section 3 reviews the residential forecast. The approach taken is to separately describe
and then critique each component of the forecast.
•
Section 4 reviews the non-residential forecast.
Box 1: Scope of this review
The work will require peer review of two components:
1.
The residential and non-residential potable water forecasts and the modelling
approach adopted to develop them for the 2020 Price Submission by end March
2019.
2.
The residential and non-residential water forecasts and the modelling approach
adopted to inform strategic metropolitan water planning and internal asset plans by
end of June 2019.
This is to include a summary of:
•
the overall approach and implementation of the model
•
model diagnostics, if applicable
•
reasonableness of approach and key assumptions required to forecast demand
•
hind cast and replication of the results from the data sets used to produce the
forecast (if desired).
Page 1
Commercial in Confidence
2. Background and context
2.1 The need for forecasting requirements
2.1.1 Uses for demand forecasts
Sydney Water is interested in forecasts of water demand for a number of reasons. These
include:
•
as an input into the pricing determination process
•
support of WaterNSW’s forecasting of long term water requirements, and
•
other internal uses including business and financial planning.
The primary requirement for the STDF model and focus of this report is to provide a five
year forecast to July 2024 as an input into IPART determinations of usage and service
charges.
Forecasts of water use are a key component of this process. The pricing determination
process has broadly involved determining:
•
a revenue target based on forecast capital and operating costs
•
a volumetric price for water over the revenue period1
•
a forecast level of revenue from usage charges, calculated as the product of the demand
forecast and the volumetric price, and
•
fixed and other charges, calculated to address any revenue gap not recovered from
usage charges.
As such, the annual demand forecast effectively determines the fixed charges. Revenue from
water use charges is in around 1.1 billion dollars per year2 and so the cost to Sydney Water of
a one per cent deviation from forecast demand is approximately $11 million per year.
The annual demand forecast has a minor impact on Sydney Water’s total costs and therefore
revenue requirement.3 The key implication of the forecast is to affect the revenue obtained
from Sydney Water’s customers and as a result the profit that is returned to its owners (being
the State Government).
1 Recently this has been set to estimate the long run marginal cost of supply.
2 In the 2017 financial year, revenue from water usage charges was $1,116 million (Source: Sydney Water
2016–17 Annual Report).
3 The forecast costs of water purchases and water treatment is based on the demand forecast so there is a
small impact on the (forecast) revenue requirement. A minor exception is that the forecast may have an
impact of the expenditure on programs to meet water use targets. Sydney Water has some variable costs (e.g.
treatment and water purchasing); however the
forecast (as opposed to actual use) will not materially influence
these costs.
Page 2
Commercial in Confidence
2.1.2 Requirements of the forecast
Given the context above, for the purposes of the IPART pricing determination the key
forecasting requirements might be summarised as to:
•
Provide a forecast—and an assessment of uncertainty with the forecast—for demand
for each financial year over a four-year period (from 2020–21 to 2023–24). As the
forecast was prepared in October 2018, in-effect a six-year forecast (from 2018-19 to
2023-24) was prepared.
•
Ensure that the forecasting approach and drivers of the forecast are clear and
transparent for the benefit of key stakeholders including IPART, senior management
and the board.
2.2 Sydney Water’s approaches to forecasting
Prior to 2011, Sydney Water had used a ‘top down’ approach to forecasting demand. This
approach started with an assumed baseline level of demand for water in terms of litres per
capita per day (LCD) and made adjustments for factors such as water conservation measures.
Concerns with that approach led to a more sophisticated approach being introduced in 2011
(the 2011 Forecast, for the purposes of forecasting demand for the July 2012 to June 2016
pricing determination). The new approach involved separate forecasts for residential and
non-residential segments using billed customer data and an estimate of leakage and other
components of non-revenue water.
2.2.1 Residential segment
For the residential segment, econometric models were developed by Dr Vasilis Sarafidis
(then at Sydney University) to estimate the influence of a number of variables including
price, weather, season, restrictions and demand management programs. The models were
estimated using data from the period July 2005 through to December 2011, a period during
which there was a significant drought, restrictions and demand management measures and
significant price changes.
The 2011 Review found the approach to be reasonable relative to alternatives. The same
broad approach was used again in 2014 in forecasting for the 2016 Price Determination
Period. The key changes were use of a different set of data, which in turn prompted different
modelling specifications, and a revised approach for applying the models. I reviewed the
2014 forecast and concluded the adopted approach appeared to be adequate and robust for
the purpose of the forecast. I understand that a range of models were examined and that the
most reasonable modelling specifications were selected.
The new residential demand forecast follows a similar approach to 2011 and 2014. Dr Vasilis
Sarafidis was again employed to develop econometric models of residential demand. The
models used by Dr Sarafidis and the approach used to apply these models is also similar.
The key changes (elaborated in the following sections) are:
•
a slight change in time period used
•
the inclusion of separate variable for upward and downward price movements, and
•
a change in the weather variables used.
Page 3
Commercial in Confidence
2.2.2 Non-residential segment
For the non-residential segment, the forecasting approached adopted in 2011 involved
further segmenting non-residential demand into the top-6 users and 7 other sub-segments.
Regression models were used to estimate average demand in each of the sub-segments,
which were combined with forecasts of property numbers to develop a forecast. I reviewed
the approach when it was proposed in 2011 and found it to be reasonable relative to
alternatives.
In 2013 the non-residential demand forecast was re-examined for the purposes of developing
a long-term demand forecast (LTDF) for potable water. The LTDF required forecasts for
each of Sydney Water’s 13 delivery systems. This non-residential forecast for the LTDF (the
2013 Forecast) adapted the approach of the 2011 Forecast but was updated with additional
data and with some modifications applied to the regression models.
In 2014/15 the 2013 Forecast was used for the submission to IPART for the July 2016 to
June 2020 pricing determination period (the 2015 Forecast). In response to an IPART
request, the forecast was refined to include an adjustment for an upcoming price change.
The forecast being reviewed (the Current Forecast) is the 2015 Forecast with some
additional adjustments.
Page 4
Commercial in Confidence
3. Review of the STDF for
residential demand
3.1 The overall approach to residential
demand forecasting
3.1.1 Description
The broad approach to forecasting the residential demand involves developing models of
billed household residential consumption based on 26 quarters of historical data4 and using
these models to forecast future demand for each household.
The approach has involved three stages:
1.
Developing models of changes in water demand
Econometric models were developed that describe how—for 33 different property
segments—household demand varies with price, season and weather. The output of
this stage is a series of parameter estimates of how household demand (measured as the
log of consumption) varies by factor.
2.
Calibrating the models and forecasting average demand by household type
Sydney Water calibrated the models to match actual household demand by household
and applied them to forecast demand by existing households and new households by
household type for each quarter to June 2025.
3.
Aggregating the forecasts using projections of dwelling numbers.
In the final stage Sydney Water combined the forecast of average demand by household
type with forecasts of dwelling numbers to estimate an aggregate level of demand by
quarter by system to June 2025.
3.1.2 Assessment of broad approach
In my opinion, the broad approach is reasonable given the lack of practical alternatives. Of
note there does not appear to be a reasonable alternative to the use of
billed household data.
Daily bulk water data is available, which might enable a more refined analysis of the short-
term impacts of weather. However, it is aggregate data that combines all customer segments
(residential and non-residential) and non-revenue water (which constitutes around 10% of
bulk water). Using this data would limit Sydney Water’s ability to analyse the impact of
changes in the number of properties (by property type) and how different customer
segments respond to changes in the weather.
4 Jul–Sep 2011 to Oct–Dec 2017.
Page 5
Commercial in Confidence
3.2 Econometric model for the residential
demand forecast
3.2.1 Overview of the model
Models of individual average daily household water consumption were developed and
estimated by Dr Vasilis Sarafidis from Monash University using data prepared by Sydney
Water. A description of the modelling methodology and results is provided in a paper
(hereafter the Methodology-Results Paper)5 by Dr Sarafidis. Following queries I raised, Dr
Sarafidis undertook some additional analysis that involves examining the impact of trend
values and expanding the estimation period (hereafter, the Trends Paper).
The key aspects of the residential demand modelling approach are:
•
Data:
Models were estimated using household billed water consumption over 26 quarters
from July 2011 to December 2017
Additional data was collected on properties (including property type, tenancy and
lot size) and weather variables on a five by five kilometre (km) grid
•
Clustering. In total 31 different models were run based on a different property types
and characteristics (BASIX status, availability of recycled-water and tenure) segmented
into different property size clusters.
•
Model structure. The models all followed the same broad structure, involving:
a semi-log form, whereby consumption is expressed in logarithmic (log) form and
other variables were expressed in level terms
a one period lag of consumption (specifically log of average daily consumption)
other explanatory variables including price, weather variables and seasonal dummy
variables.
The modelling approach is largely consistent with the approach used in the previous STDFs.
The key changes from the previous STDF are:6
•
a change in the estimation period (the time period over which demand is estimated)
and—resulting from this—a change in the variables that are used
•
allowing for different responses to price decreases and prices increases (in response to
concerns by IPART)
•
consideration of a broader range of weather variables
•
participation in demand management programs was dropped as a segmentation and
explanatory variable.
5 Full title ‘The Econometric Methodology and Results for 2018 Demand Forecasting Project for Sydney
Water Corporation’, 13 November 2018.
6 The Methodology-Results Paper also lists that ‘Prices are exogenous’ as a change to prior studies. I
understand that this change occurred in developing the previous STDF.
Page 6
Commercial in Confidence
Data and model
The general model applied to all clusters is given in the structural equation Box 2 below. The
model is similar to that used in the 2014 study. The key modifications are to the independent
variables being:
•
inclusion of additional weather variables
•
inclusion of method to measure a separate impact for price increases and decreases, and
•
removal of participation in demand management programs.
Box 2: General model used
The structural equation is of the form:
ln = × ln
+
×
× ∆ + ×
× (1 − ∆ ) +
×
ℎ , +
×
, +
Where:
ln represents the natural logarithm of average daily consumption (c) for
household
i in time period t
is a measure of the usage price for filtered water faced by each household
ℎ , is a set of weather variables (see Table 1)
, is a set of seasonal dummies. The base is set to be spring
∆ is equal to 1 if ∆
< 0and 0 otherwise
, , , and are parameters to be estimated
= + is an error term reflecting that variation of household demand can
deconstructed into a time invariant (and household specific) component ( ) and a
time variant component .
The time periods used reflect the billing periods for each household in the sample. As the
date of the meter reads for each household are slightly different, the time periods for each
household are slightly different. An implication of this is that variables that might be
considered as common for each household (e.g. price, weather and season) are modified to
reflect the timing of the meter reading. Thus, for example, the price variable reflects the
average price faced by a household over the period since the last meter read.
Weather variables are also household specific because of the different meter reading dates
and the fact that weather conditions can vary significantly over Sydney Water’s area of
operations on the same day. The latter variation is captured by using gridded weather data (in
5x5 km grid-blocks) obtained from the Bureau of Meteorology (BoM).
The weather variables used are listed in Table 1 below. Each property was assigned a grid
cell. Sydney Water then attached the daily weather data from that cell and aligned it with the
Page 7
Commercial in Confidence
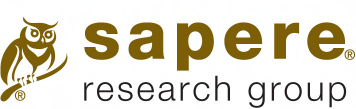
meter-reading dates for the property to calculate the weather variables. Variables described as
an ‘anomaly’ refer to the difference between the observed value and the average value over
the 30 years to December 2017.7
Table 1: Weather variables used and considered
Whether used
Variable
Used in current and prior
•
Average maximum temperature anomaly
(2014) study
•
Average daily rainfall anomaly
•
Average daily (pan) evaporation anomaly
•
Number of days with max temperature > 30C
•
Number of days with rainfall > 2mm
•
Season
Used in current study but
•
Number of days with max temperature > 40C
not in prior study
•
Longest run of days without rainfall
•
Longest run of days with rainfall ≤ 1mm
Other variables considered •
Number of days with max temperature > 35C
but not used
•
Max run of days with rainfall ≤ 2 mm
•
Max run of days with max temperature > 30C
•
Max run of days with max temperature > 35C
•
Max run of days with max temperature > 40C
3.2.2 Assessment
Given the data available, the econometric approach appears reasonable. I see no reason to
expect that the model structure employed could be materially improved upon. Nevertheless,
some additional commentary is provided below.
Use of clustering
The approach of clustering appears reasonable. Given the large volume of data available
there appears no downside to estimating different models for different clusters (other than
the production of a large volume of results).8 The approach used (described in the
Methodology-Results Paper) to undertake the clustering appears reasonable.
7 For example, for a billing period 1 May – 30 July 2017, the average daily rainfall anomaly is calculated as the
average daily rainfall for this period less the 30 year average.
8 Conceivably, the factors that were used in clustering (i.e. the property-type and size) could have been used in
a model specification; however, this would have led to a significantly more complicated analysis.
Page 8
Commercial in Confidence
The use of a semi-log structure
The models are estimated using a semi-log form, whereby the log of consumption is used
and all other variables are expressed in level terms.
This approach is consistent with the previous STDF models. I understand that alternative
approaches (e.g. log-log and level-level) were not examined in this study but were considered
in previous studies and not shown to result in any material differences.
As discussed in previous reviews, I have no material concerns9 with the use of log
consumption as measure of demand. The use of double-log (or log-log) was justified in the
Methodology-Results Paper on the basis that:
•
a double-log specification imposes a requirement of a constant elasticity, which is
viewed to unlikely be realistic, and
•
a double-log form leads to coefficients that are more difficult to interpret.
I have no reason to believe the model structure employed could be materially improved
upon.
The use of lagged consumption
I had considered the use of lagged consumption in my 2014 Review. I believe these
comments are still appropriate. In that review I wrote:
A key benefit of using lagged consumption is to enable modelling of short and long-
term effects of changes in key variables. This has been particularly important in
estimating the effect of price changes, which as discussed are not a material
consideration for the period of estimation. The use of lagged consumption is less useful
(and rather adds complexity) for the modelling of the impact of other variables. For
example, it is not expected that the weather and the seasons will have both short and
long-term effects. Using lagged consumption may also improve the model estimation10
and improve the forecasting in the short-term.11
The key downside of using lagged consumption is that it adds complexity to the
modelling and application of the models. Given the existence of the prior models and
the established procedures this does not appear to be a significant cost.
In summary, it would be simpler and there is potentially little downside to not using
lagged demand in the modelling. Nevertheless, aside from the complexity I have no
material concerns with the approach used.
9 The key downside of using log demand (as opposed to a level measure of demand) is that it adds complexity
to the modelling process. It means that an adjustment is required to undertake the forecast to reflect that
estimation error (in level terms) is skewed.
10 That is, improve the estimation of other parameters in the model.
11 In this case, this benefit is limited as the forecast is for a large number of quarters over which time the
influence of the most recent recorded (i.e. actual) level of consumption will be very small.
Page 9
Commercial in Confidence
Time period used
The time period of the data is of some interest. The 2014 STDF used data beginning from 1
year earlier (i.e. including the 2010/11 year) than the current model.
Typically for modelling purposes there is an interest on using more, not less, data. However,
there are a few concerns for Sydney Water in using the older data.
•
The older the period, the less relevant the data becomes. This is because dwellings
change ownership undertake renovations etc.
•
The 2010/11 period was not long after the period that restrictions was removed and
consequently the data was likely to be influenced by bounce-back in demand
•
The data is limited to properties for which there was billing data for the full period. The
more quarters of data that are used, the fewer properties can be used.
I understand that for these reasons, Sydney Water’s preference was to use even fewer periods
and that the 2011/12 year was included because it was a period that incorporated some more
extreme weather periods.
A concern with excluding the 2010/11 year is that it is the period with the most significant
price increase in the available data. In response to this concern, some testing was conducted
using the additional 4 quarters from in 2010/11 for the largest clusters. This testing
(documented in the Trends Paper) included inclusion of specifications with time trends
(both linear and quadratic).
The most notable effect of using the additional year of data is a reduction in the estimated
impact of price. However, this result may reflect the timing of a price increase with a
bounce-back in demand following a lifting of restrictions. The models that use a quadratic
time-trend are consistent with this hypothesis. Furthermore, I understand the implied price-
elasticities of the base model (i.e. excluding the additional 4 quarters) are more consistent
with the previous estimates of the price-elasticities. In light of the results—given the risk of
bounce-back influencing the 2010/11 data—I believe it is not unreasonable to exclude the
2010/11 data from the estimation period.
Explanatory variables
Price
The price variable used is calculated as the nominal price divided by an annual measure of
the consumer price index (CPI). The price by financial year used is provided in the table
below.
Table 2: Price variables used
Financial year
2010/11 2011/12 2012/13 2013/14 2014/15 2015/16 2016/17 2016/17
Nominal price
2.012
2.103
2.13
2.168
2.232
2.276
2.000
2.040
Real price
2.292
2.337
2.308
2.289
2.311
2.322
2.000
2.000
(base 2016/17)
Real price change
0.045
-0.029
-0.019
0.022
0.011
-0.322
0
Page 10
Commercial in Confidence
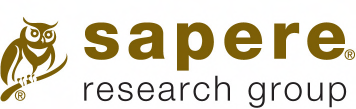
The nominal usage price in each financial year is, itself, based on a real price set for each
pricing determination period by IPART multiplied by a CPI measure. The CPI measure used
by IPART differs to that used by Sydney Water in that:12
•
IPART used the CPI as at the end of March (Sydney Water use the CPI as at the end of
June). and
•
IPART used the national CPI figure, whereas Sydney Water used a Sydney-specific
measure.
Consequently, the variations in price are a result of changes in the real prices determined by
IPART, which occurred in July 2013 and July 2016 and other differences in the CPI
measurement.
The estimates of the impact of price have some relevance to the forecast. I understand the
current forecast assumes no change in the real price; however I assume there is a risk that
this may change. Furthermore, the current real price differs materially to the average real
price that applies in the calibration period. Consequently, a change in the estimated impact of
price would change the calibration and the forecast.
As shown in Table 2, the real price change was significant in 2016/17 but was small in all
other years. In my opinion, given the small variation in price movements (and the lack of
attention given to price changes), care should be taken in interpreting the price coefficients
as there is a risk that the results are reflecting some other variation that is not captured in the
other explanatory variables.
Nevertheless, I expect the risk associated with estimating the impact of price is low. The
modelling results for price do not appear unreasonable as they are broadly consistent with
expectations and previous estimates.13 Furthermore, the variation in price for the forecast
(which is current price) to the average of the estimation period is around 10 per cent, which
is material but not overly significant.
The modelling results suggest that the response to price is not asymmetric (i.e. the demand
response to price increase is similar to a price decrease). For small prices changes this does
not seem unreasonable.
The weather measures
In my opinion the use of the weather measures in the modelling is reasonable.
Weather is a major factor in determining water demand. Not properly accounting for the
weather could lead to inaccurate forecasts, particularly if the forecast weather differs to that
12 In determining real prices for the forecasting study, Sydney Water used the Sydney CPI as at the end of June.
The CPI used by IPART for determining nominal prices is defined in Section 1.2 (Consumer Price Index) in
the Schedule on ‘Definitions and interpretation’ in IPART Price determinations (in Schedule 8 in 2012 and
Schedule 9 in 2016). In summary it:
•
means the consumer price index, All Groups index number for the weighted average of eight capital
cities as published by the Australian Bureau of Statistics
•
is measured as at the end of March in each year
Note: The 2012 determination also stated the CPI would be ‘adjusted by subtracting a Carbon Price Impact
which is an estimate of the impact of the Carbon Price on the CPI.’
13 I calculate the implied short-run price elasticity estimates for the owner-occupied single-dwellings to range
from -0.04 to -0.15 and are more significant for large block sizes (as expected).
Page 11
Commercial in Confidence
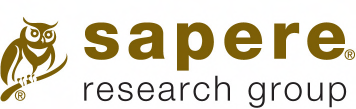
used in the estimation period. Consequently, it is appropriate that—as has occurred—
significant effort is expended in selection of the weather variables.
I have no material concerns with the functional form of how the weather variables were
introduced. As noted in the 2014 review, two matters deserve comment:
•
The functional form used to model the relationship between consumption and weather
is log-linear; that is, the models assume the log of consumption changes linearly with
temperature and rainfall. This implies, for example, a one unit increase in average
rainfall has the same multiplicative effect on demand regardless of the overall volume of
rainfall. The true relationship between consumption and weather is likely to be more
complicated; for example, the effect of additional rainfall on log consumption may be
less significant for high levels of rainfall. Nevertheless, I understand that different
functional forms were tested as part of the 2014 review and the form chosen was
selected as most appropriate.
•
The model includes the implicit assumptions that the marginal effect of weather is
constant regardless of the season. This appears surprising. In previous work (reviewing
Sydney Water’s climate correction model, which uses bulk water aggregate data) I found
evidence that changes in the weather has more of an effect in summer and spring than
winter. Nevertheless, I understand that the interaction of season and weather variables
was tested (in prior STDF) and found not to be significant.
Time trend
As with all regression modelling there is a risk that there are some other unobserved factors
influencing demand that are not included in the model. Such factors may bias the forecast if
they are trending and continue to do so following the estimation period.
To address this concern, some analysis was undertaken to test whether there were any
trends. The test involved examining re-estimating the models with the linear and quadratic
trends applied. The tests were conduct for the 6 clusters within ‘owner-occupied, pre-BASIX
single dwellings without recycled water’ segment. The results of this analysis—documented
in the Trends Paper—suggest there may be small downward trend in some of the clusters
examined.
Once possible reason, (based on discussions with Sydney Water) is that there is an increase
in the water efficiency of existing single dwellings as a result of renovations (e.g. as people
renovate bathrooms etc). I assume this issue would be more significant for single dwellings
than units.
If such a downward trend exists and continues, then there is a risk that the forecast—which
does not allow for such a trend—is too high. However, this risk seems very small. As
illustrated in (see section 3.5 Modelling results) there is evidence of a slight downward trend
but it is very small relative to other variation.
Other variables
The variable ‘participation in demand management programs’ was removed. This is
reasonable because there was—I understand—no significant changes in the participation in
demand management programs during the estimation period.
Page 12
Commercial in Confidence
3.3 Calibration and forecasting
3.3.1 Overview of the process
The calibration and forecasting stage involved four steps.
First, a calibration process was performed that involved estimating a household level
constant (the value , referred to in Box 2 on page 7) for all existing households with at
least 5 quarters of consumption data.14 This was achieved by applying the appropriate
regression models to each household and setting the household specific constant as the
average difference between model estimates and the observed values. This calibration
process is performed over the same period used to estimate the regression models (July 2011
to Dec 2017). For properties connected after July 2011 Sydney Water used all available data
up to Dec 2017.15
Second, a forecast of demand is made for every household in the sample using the
appropriate regression equations. For the forecast, the price is assumed to be constant (i.e.
no change in real prices).
The forecast weather variables are based on analysis from NSW and ACT Regional Climate
Modelling (NARCliM) Project (see Box 3 below).16 The NARCliM outputs were used as
follows:
•
Selection of forecasts. The NARCliM projections result in 12 models and three
different time periods. The median demand forecast derived from the different
NARCliM models for the near future time-period (‘2020 to 2039)’ was used.
•
Translation of data variables. The NARCLiM output for daily temperature and rainfall
were translated into the weather measures used in the regression model (i.e. average
daily rainfall, number of days greater than 30 C etc.). Pan evaporation is not an output
produced by NARCliM. Instead, pan evaporation was estimated based on temperature
and rainfall using a model developed by Dr Adrian Barker at UNSW.
•
Geographic matching. NARCliM outputs results on a 10x10 km grid. The Climate
Change Research Centre and UNSW which carried out the modelling for NARCliM
interpolated the results for Sydney Water to a 5x5 km grid consistent with the grid used
for the BoM data.
Box 3: NARCliM Modelling Overview
The NARCliM projections have been generated from four global climate models (GCMs)
dynamically downscaled by three regional climate models (RCMs).
14 This is around 97–98% of households.
15 This calibration is carried out on ‘apportioned’ consumption. It corresponds with the measure of
consumption that is required for converting the demand forecast to revenue. The apportioning is carried out
consistently with the method that is used to apportion meter readings that cover periods with different water
usage prices. It is readily available for all properties from Sydney Water’s Business Intelligence system.
16 The NARCliM Project is a research partnership between the NSW and ACT governments and the Climate
Change Research Centre at the University of NSW.
https://climatechange.environment.nsw.gov.au/Climate-projections-for-NSW/About-NARCliM/
Page 13
Commercial in Confidence

The four GCMs (MIROC, ECHAM, CCCMA and CSIRO Mk3.0) form part of the World
Climate Research Program's (WCRP) Coupled Model Inter-comparison Project phase 3
(CMIP3) models, which were used by the Intergovernmental Panel on Climate Change
(IPCC) for its Fourth Assessment Report. See the NARCliM Model Selection for more
information on how the models were selected.
The Weather Research and Forecasting (WRF) model, a dynamical regional climate mode,
was used to downscale projections from the four GCMs. WRF has been demonstrated to
be effective in simulating temperature and rainfall in NSW and provides a good
representation of local topography and coastal processes. It was jointly developed by
several major weather and research centres in the United States and is widely used
internationally.
Three physical configurations of WRF were run with each of the four separate GCMs, for
a total of 12 runs, producing a 12 model ensemble. The 12 models were run using a single,
representative emissions scenario: the IPCC high emissions scenario A2. The 12 models
were run for three time periods: 1990 to 2009 (base), 2020 to 2039 (near future), and 2060
to 2079 (far future). A reanalysis dataset is also available for the period 1950 to 2009.
The NARCliM models generate data for more than 100 meteorological variables.
Source: https://climatechange.environment.nsw.gov.au/Climate-projections-for-
NSW/About-NARCliM/
The third calibration-and-forecasting step involves an adjustment to convert the estimated
demand into level terms (as opposed log-demand). The approach adopted involved applying
the first method referred to in Box 4 below and then applying a variant of the second
method to correct for any discrepancies.
The fourth step involved using the quarterly forecasts for each individual property, to
calculate average demand for each property type and system. Properties are grouped by 4
property types (single dwellings and dual occupancies, townhouse strata units, vertical strata
units and flats), 2 BASIX statuses (pre or post BASIX) and 11 delivery systems. The results
for the individual properties are then averaged by these groups resulting in 8 forecasts of
average demand for each system.
These above steps are implemented using SPSS software. Although SPSS is a statistical
package, no statistical analysis is undertaken. Rather SPSS is used for its data management
capabilities.
Box 4: Adjustment because the dependent variable is in log form
For the purposes of forecasting, a value is required in level (i.e. not log) terms. Log values
can be transformed into level values by exponentiating them; however, an adjustment is
required to prevent a bias being produced by exponentiating the error term in the
modelling equation.
For example, assume the demand equations is rewritten as:
ln =
+
Page 14
Commercial in Confidence

Where ln is the natural log of average daily consumption, X is a series of regressors, a
vector of parameter values to estimate and an error term. Using “^” to denote predicted
terms and “E” to denote the expected value then
ln = (
+ ) =
since E( ) = 0
However, the predicted value of c is not so easily obtained. It is the case that:
̂ ≠
. Rather ̂ =
a where a = ( )
There are two common methods to estimating a :
1.
If is normally distributed with mean 0 and variance then ( ) = exp( /2)
and so an estimate is simply a = exp( /2)
2.
An estimate of a can be obtained from the single coefficient obtained from
regressing
c on
(ln ) without an intercept.
Source: Adapted from Wooldridge (2003, Section 6.4).
3.3.2 Assessment
In my opinion the calibration approach—which largely follows what was done for previous
STDF—is reasonable.
The forecasting step includes the most significant assumptions and therefore presents the
most significant risk to forecast.
The weather is the greatest source of uncertainty and influence in forecasting the future
demand of water. The most significant assumption is that weather experienced in the next 5
year period is best estimated by the median result of the NARCliM scenarios. I have no
cause to question this assumption.
The forecast includes others assumptions including:
•
The implicit assumption that behaviour identified in the recent past will be
representative of the future
•
Any reduction in use due to improvements in water efficiency (or installation of
rainwater tanks) in existing dwellings will be small or offset by other trends in usage
•
There is no change in the real price.
Given the calibration step, the sensitivity of the results to these assumptions is likely to be
small.
As noted in the previous review, one downside with the approach of generating the system-
wide forecasts from a sum of individual property forecasts is that no system-wide model is
produced that illustrates the sensitivity of demand to variations of key variables (e.g. price
and weather). However, this issue could be addressed by using a numerical approach that
involves calculating how the system-wide results change in response to small changes in the
input variables. I understand Sydney Water is considering such an exercise (when it has
capacity).
Page 15
Commercial in Confidence
3.4 Aggregation of the forecast
3.4.1 Overview
The final stage involves aggregating the forecast by (the relatively straight forward process
of):
•
multiplying the average forecast demand by the forecast number of properties by
property-type
•
summing the property-type forecasts to produce an aggregate.
The forecast number of properties in each grouping is obtained by applying estimates of
dwelling growth provided by the Department of Planning and Environment (DPE) to
Sydney Water counts of properties. Sydney Water uses DPE’s forecast to develop forecasts
for each area of operations and then split into single and multi-dwelling forecasts based on
observed patterns. These forecasts are further split into the segments again based on
observed historical patterns.
In apply the dwelling forecasts Sydney Water assumes that the each new single-dwellings in a
system have the same average lot-size as the existing BASIX properties in that system.
3.4.2 Assessment
I understand from Sydney Water that the current number of residential properties at a point
in time (to get a starting point for the forecast) can be counted accurately.17 The process of
aggregation is relatively straight-forward that relies on DPE’s forecasts and an assumption of
the demand of new properties. I have no concerns with the aspect of the process.
3.5 Modelling results
The aggregate modelling results are summarised in Figure 1 below, which shows the actual
results (in orange) and in the hindcast and forecast (in blue). As shown in the figure, there is
negligible difference in the forecast (which starts from December 2017) by year. The small
differences that exist are attributable to the effect of lagged consumption (which has is
observable in quarters 3 and 4 of 2017–18) and that every fourth year is a leap year.
17 I understand that, due to reclassifications of property types, it can be more complicated to determine
historical counts.
Page 16
Commercial in Confidence
Figure 1: Hindcast/forecast plus actual for all residential dwellings
Single and multi-dwel ings - pre + post-BASIX
120,000
100,000
80,000
uarter 60,000
L per Q
M 40,000
20,000
0
HIND/FORECAST
ACTUAL
The differences between the hindcast and actual (the hindcast errors) are shown in Figure 2
below. The hindcast error in any one quarter can be substantial (in excess of 4% of the
actuals). The figure also shows the trend line (the red dashed-line) associated with errors.
Consistent with the findings of the Trends Paper, there is evidence of a slight downward
trend. However, the trend—when viewed alongside the hindcast errors—appears very small.
The results suggest that the models do a reasonable job of predicting demand; however, it is
difficult to make an assessment of the results given the limited number of observations.
Page 17
Commercial in Confidence
Figure 2: Relative Hindcast error
Relative hindcast error - Single and multi-dwel ings - pre + post-BASIX
5%
4%
3%
2%
1%
0%
-1%
-2%
-3%
-4%
-5%
1 2009-10
2 2009-10
3 2009-10
4 2009-10
1 2010-11
2 2010-11
3 2010-11
4 2010-11
1 2011-12
2 2011-12
3 2011-12
4 2011-12
1 2012-13
2 2012-13
3 2012-13
4 2012-13
1 2013-14
2 2013-14
3 2013-14
4 2013-14
1 2014-15
2 2014-15
3 2014-15
4 2014-15
1 2015-16
2 2015-16
3 2015-16
4 2015-16
1 2016-17
2 2016-17
Q
Q
Q
Q
Q
Q
Q
Q
Q
Q
Q
Q
Q
Q
Q
Q
Q
Q
Q
Q
Q
Q
Q
Q
Q
Q
Q
Q
Q
Q
relative error
Linear (relative error)
3.6 Summary and conclusion
At a high-level the residential forecast involves forecasting aggregate demand as a product of:
•
forecast average demand by household type based on models by property segment that
estimate how household demand changes with changes in weather, season and price
•
projections of dwelling numbers by household type using dwelling growth numbers
supplied
The forecasts of average demand (with minor qualification) do not change by year. In effect,
the main use of the models is to account for the influence of weather.
The underlying modelling of demand is quite complex as the models estimate log-demand
and involve a lag in demand. Nevertheless, the modelling and forecasting procedure used is
consistent with how it has been previously conducted and the results of the model are
transparent.
Given the available data, I conclude the modelling approach is adequate and robust. Some
minor qualifications are discussed in the body of this report. Most significantly, I note some
evidence of a slight downward trend in demand by some single-dwelling segments (which
may be consistent with improvements in water efficiency). If such a trend exists and persists
then the forecast may overstate demand. Nevertheless, the aggregate results suggest that this
risk is very small.
The main source of uncertainty in the forecast is the weather. Since the previous STDF
Sydney Water has attempted to reduce this uncertainty by investing in improvements in the
modelling and forecasting of the weather.
Page 18
Commercial in Confidence
4. Review of the STDF for non-
residential demand
4.1 The overall approach to non-residential
demand forecasting
4.1.1 Summary of approach
The non-residential forecast (Current Forecast) is best simply described as:
•
the 2015 Forecast of non-residential demand (most of which was conducted in 2013)
•
modified with some adjustments and improvements.
The 2015 Forecast was developed using 66 models to separately estimate demand for 8
segments across 11 different systems.18 Forecasts by segment/system were based on a
forecast number of properties multiplied by an estimated average demand per property,
which was largely assumed to be constant.
The recent adjustments include updates to the forecast number of properties (which was
applied to the underlying models) and an adjustment to the aggregate forecast.
An overview of these two components is below.
The non-residential forecast is combined with a forecast of ‘other billed consumption’ to be
a ‘non-residential
plus other forecast’.
4.1.2 The 2015 forecast
The 2015 Forecast involved:
1.
segmentation across 8 segments being the top-6 users and 7 other segments that are
based primarily on the billing property type and 11 delivery systems
2.
forecasting average demand by segment. This involved by segment/system:
(a) data preparation, including calculating a deseasonalised average daily demand
(b) analysing demand and property numbers to assess trends
(c) applying a regression model to correct for weather and trends
(d) estimating the future average demand based on the most recent 3 years.
3.
estimating a trend in property numbers—generally based on a simple linear trend.
4.
calculating demand by segment and in total—creating a demand forecast based on
average demand and forecast property numbers. This involved for each segment
18 Only 66 models were required due to some aggregations of systems and segments across systems.
Page 19
Commercial in Confidence
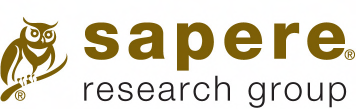
multiplying the forecast property numbers by the average demand per property and
aggregating the forecasts.
5.
making an adjustment for price movements—making an adjustment to total
demand for price movements.19
6.
running the forecast under using long term average weather conditions.
7.
making an additional adjustment for WICA sites.20 WICA sites are classed as non-
residential customers; however, their usage is forecast using the residential model based
on the projected number of residential properties on the sites. WICA sites were not a
consideration when the non-residential model was first developed.
4.1.3 Current Forecast adjustments and modifications
An analysis that compared actuals to the 2015 non-residential Forecast found that the 2015
Forecast tended to:
•
under forecast demand, and
•
under forecast the extremes of weather.
In response to these findings Sydney Water, has corrected an error in the previous forecast21
and has proposed as series of adjustments to develop the Current Forecast. These are
1.
A modification to the price adjustment step
2.
An adjustment for faster-than-expected population growth
3.
Addition of an extra 1.1 GL/year to the 2015 forecast (~1% of non-residential
demand).
The first two adjustments are applied to the model for each segment. Adjustment 3 is made
against the total.
The approach to forecasting weather conditions was also changed. Instead of using long-
term average weather conditions (see step 6 in Section 4.1.2 above), the Current Forecast
uses a more sophisticated approach involving modelled weather scenarios (see Section 4.2.2)
19 This final step was added in response to an IPART concern there would be a demand response to a forecast
price decrease.
20 A ‘WICA site’ refers to a site serviced by a company licenced under the Water Industry Competition Act.
21 I understand that Sydney Water accidentally introduced a mistake into the 2015 Forecast—equivalent to a 3
GL/ year underestimate—whereby an assumption for “Other” properties was recorded as 1 GL/year
instead of 1 GL/quarter.
Page 20
Commercial in Confidence
4.2 Details of the forecast
4.2.1 Aspects of the 2015 forecast
The segmentation
The segmentation is on the basis of property type code, of which there are around 54 types
for non‐residential properties. Three of the six segments are based on the most common
property types being Commercial, Industrial and Commercial and Industrial Strata Units.
The other segments have been grouped according to size and similarity of characteristics.22
Table 3: Non-residential segments
Average
Properties
Category
annual use
Description / Note
(2018/19)
in forecast
Commercial
43 GL
30,810
Commercial property type plus Hotels
EDC
33 GL
682
Every Drop Counts participants
Government,
27 GL
14,775
Including government organisations; public
Institutional
reserves, hospitals, universities, private
and Other
schools and hospitals, church properties
and clubs
Industrial
23 GL
15,689
Industrial property type only
(excluding top 6)
Non-
11 GL
53,415
Industrial Strata Unit and Commercial
residential
Strata Unit property types
units
Top 6
7 GL
6
The 6 largest users (All industrial
properties)
Agricultural
5 GL
2,050
Comprises farms, nurseries, orchards etc
Standpipes
1 GL
974
Portable hydrants
22 The key stated principle in grouping properties was that ‘the resulting categories should have distinct
historical consumption patterns or some other distinguishing feature that is relevant for forecasting
purposes.’ (2011 Review paper).
Page 21
Commercial in Confidence
Forecasting average demand
The process of forecasting average demand involved a number of steps.
The first step involved calculating a deseasonalised average daily demand by month from July
1989 to June 2012. This involved:
•
estimating daily demand from quarterly meter readings by calculating the average daily
demand between two meter readings and aggregating to obtain daily, and ultimately,
monthly totals23
•
deseasonalising the monthly demand totals (using a ratio to moving average method).
The second step involved plotting average demand, property numbers and total demand for
each segment and assessing trends against other known changes and features of the property
type. From this process a regression model was developed to determine a predicted average
daily deseasonalised demand (see Box 2) below. The same basic structure was used for all
segments and systems. The regression model, estimates demand against rainfall, temperature
and evaporation over the period 1989 to 2012 plus a series of half-yearly dummies.
The final step was to calculate the average demand per property, which was the average of
the weather corrected model predictions for the most recent 3 years (i.e. Jul 2009 to June
2012).
Box 5: Regression model used
The basic model used for forecasting each segment involves estimating, by segment,
month/ year, the deseasonalised average daily consumption per property. The
deseasonalised average daily consumption is calculated from average daily consumption
for a month using a monthly seasonal factor.24
The non-residential demand equation is described in Equation 1 below.
Equation 1
∗ = + ∆ + ∆ + ∆ +
+
Where:
•
the subscript t signifies the month/year and h the half year
•
∗ = / is the deseasonalised average daily demand in month
m corresponding
to time
t and
is a monthly seasonal factor
is average daily demand for the month estimated from meter reading data
•
∆ , ∆ , ∆ are deviations of maximum temperature, evaporation and rainfall from
their monthly average over the years of the sample.
23 The consumption for a month is estimated based on the quantity consumed during a meter reading period.
Consumption during a meter reading period is allocated on an average daily basis across months that fall in
the meter reading period.
24 Deseasonalised consumption refers to consumption achieved from dividing the monthly result by a seasonal
factor so that the average monthly result is the same for all months.
Page 22
Commercial in Confidence

•
is a dummy for each of the 37 half-year periods from Jan–Jun 1990 to Jan–Jun
2012
•
is an error term.
Estimating a trend in property numbers
Property growth was forecasted based on historical growth rates by fitting trend lines to
historical property numbers—a process which involved some judgement.25
Adjustment for price
The total non-residential forecast was adjusted upwards to account for price changes by
amount estimated as the product of:
•
the proposed priced changes at the time—specifically a real price drop of around 14 per
cent from 2015/16 to 2016/17 was proposed as part of the price determination
•
a price elasticity of around -0.13, estimated from:
recent relevant research26
multiplied by an asymmetry of price responsiveness to account for the project
price change being a decrease.27
This resulted in an increase in total non-residential demand of around 1.8 per cent.
4.2.2 Running the forecast under a range of future
weather scenarios
Prior forecasts were based on the historical weather data. In light of concerns that the
climate is changing and that historical weather patterns may be unrepresentative a project
was undertaken in conjunction with the Centre of Excellence for Climate Extremes and
Climate Change Research Centre (CCRC) at the UNSW.28 The project team used a stochastic
25 The process is described in documentation provided to me for the 2013 Review (‘Long term non-residential
demand forecasting model Draft for peer review 20 February 2013’). Trends were based on the most recent
years so as to avoid one-off reclassifications biasing trends which had appeared to stabilise. Trends lines were
assumed to be linear except for Units where a second order polynomial was fitted and the first derivative of
the function (as at July 2012) was used for forecasting.
26 An elasticity of -0.264 was assumed. The estimate was sourced from: Worthington, Andrew C, 2010,
Commercial and Industrial water demand estimation: Theoretical and methodological guidelines for applied
economics research. Discussion Papers Economics No. 2010-11. Griffith Business School, Griffith
University, Queensland, Australia.
27 The demand elasticity was multiplied by ½ to account for the common finding the price-responsiveness is
lower to price falls than to price increases.
28 A paper describing the work, which has been submitted to Water Resources Management was provided to
me. Adrian Barker, Andrew Pitman, Jason Evans, Frank Spaninks, Luther Uthayakumaran. ‘Probabilistic
forecasts for water consumption in Sydney, Australia from stochastic weather scenarios and a panel data
consumption model.’
Page 23
Commercial in Confidence

weather generator to develop 100 different synthetic weather scenarios for the period
spanning the financial years 2014/15 to 2024/25.
The non-residential forecast model was rerun using these weather scenarios. The median
result was used as the best-case forecast.
4.2.3 Recent adjustments
A comparison of the forecast results relative to the actuals between 2010 and 2017 found
that:
•
the forecast had tended to underestimate the weather effects,29 and
•
more generally under-forecast total demand.
To address the under-forecast three adjustments have been made:
1.
Removing of the price asymmetry adjustment. This has the effect of assuming that the
price elasticity is equal to -0.264.
2.
A ‘population growth’ adjustment
This adjustment involves inflating the total demand by the ratio of the most recent
population forecast to the population forecast that was available at the time the model
was developed (in 2012).
The adjustment was originally described as a ‘property growth’ adjustment. However,
in-effect, it is an adjustment for faster than expected population growth, which is
assumed to result in greater density of the workforce at non-residential properties.30
3.
A ‘final’ adjustment. Inflating the total aggregate demand by an additional 1.1 GL per
year. This is estimated as the average difference between the forecast (including the
above two adjustments) and the actuals (see discussion below) for the prior 8 years.
4.3 Assessment
4.3.1 The overall approach and implementation
The current non-residential forecast can be summarised as:
•
the 2015 Forecast (which is a modification of the 2013 Forecast) which was aggregated
from forecasts by segment and system,
•
modified to reflect a different approach for forecasting weather, and
29 Specifically it was found that “Errors tend to be correlated with weather conditions:
•
Overestimates in years with relatively low temperatures and evaporation
•
Underestimates in years with relatively high temperatures and evaporation
•
No such clear relationship with average rainfall; probably due to sensitivity to distribution over year”
30 The adjustment is described as updating the property forecasts as
=
×
where :
property forecast for
t,
actual population served where available or most recent available forecast otherwise,
: population forecast for t when the model was build and
original property forecast
when the model was build
Page 24
Commercial in Confidence
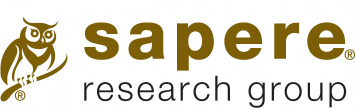
•
overlaid with adjustments made to the segment forecasts and the aggregate to account
for observed discrepancies between predicted and actual demand.
The accuracy of the forecast mostly depends on the adjustments. To-date, it is difficult to
assess these, as the final adjustment has been made to the aggregate non-residential demand
and that—due to time and resourcing constraints—there has been little analysis (other than
weather variation) on the underlying drivers of the observed discrepancies.
In meeting the accuracy objective this overall approach is not ideal. To improve accuracy, it
would be preferable to examine the performance of 2015 Forecast by segment including an
analysis of property growth and changes in average property demand. However, due to the
large number (66) of models, I understand this would be very time consuming.
As discussed below, I have a concern relating to the risk associated with changing weather.
This matter aside, I have no material concern that the implementation of the approach
would lead to a biased forecast.
The 2015 Forecast
Some assessment of 2015 Forecast is appropriate. In part because it forms the baseline from
which the adjustments have been made but also because the disaggregated approach may be
used again in the future.
I had previously evaluated the key elements of 2015 Forecast approach when I conducted a
review in 2011 and 2013. I conclude again, as in those reviews, that the broad approach—
relating to segmentation and forecasting on the basis of average demand by property type—
is reasonable. For the 2013 review I had also concluded based on a visual examination, that
the non-residential forecasts themselves appear reasonable. A summary of the key findings
from the 2013 Review on the non-residential forecast are included in the Box 6 below.
An additional potential issue with the 2015 Forecast is in how it controls for weather
variation. The 2015 Forecast models (see Box 5) include terms for weather variables and
half-yearly dummies. A risk to this approach implication is that much of the variation due to
weather may be attributed to the half-year dummies and not weather and lead to the model
being relatively poor at predicting the effects of weather.31 This may help to explain the
models relatively poor performance in predicting the variations in demand due to the
weather. Potentially this may be resolved by re-running the underlying regression models
without the half-yearly dummies and using the revised coefficients for the weather variables
for predicting future demand.
31 This is easiest to see with an extreme example. If 1-monthly dummies were used all variation would be
attributed to the dummies leaving no variation to be attributed to weather.
Page 25
Commercial in Confidence
Box 6: Key findings from 2013 Review
Assessment
Given the lack of explanatory variables that can be used, there does not appear to be a
viable alternative to the approach used of forecasting based on trends in property
numbers by segment and average demand per property.32
Given the information available, the non-residential forecasts themselves appear
reasonable. Based on a visual examination:33
•
The assumptions of average demand per property are reasonable.34
•
The trends assumed for the property forecasts appear reasonable.
•
The overall forecasts by segment by system appear reasonable.
Risks to the forecast
The approach relies on a number of subjective assessments relating to both the average
demand and the trends in property.35
There are some limitations to the data and thus the modelling. The modelling is based on
an estimated average daily use derived from quarterly billing data, which by the procedure
used results in monthly data being accumulated from billing data taken over a 6 month
period. This has a number of implications for modelling.
•
The most recent billing data cannot be effectively used until a number of months
have passed. While one would not expect this to be an issue for long-term forecasts it
does make the detection of changes in use including analysis of bounce-back, which
requires recent data, more difficult.
•
Detection of the effect of weather and seasons are difficult as seasonal and weather
variables need to be averaged over a period and matched against averaged data.
The majority of these issues are minor. The primary concern relating to the non-
residential forecasts is the lack of information on the underlying drivers of non-residential
demand and the explanatory variables that can be used to conduct forecasts.
The broad approach used is sufficiently robust for future use and the approach is
sufficiently flexible to be easily refined over time as new information becomes available.
The revised forecast for the impact of weather
As noted above, the Current Forecast uses the weather models used in the 2015 Forecast but
develops a forecast based on the median result from applying weather scenarios developed
by CCRC at the UNSW. I have no reasons to question the weather scenarios that have been
32 A challenge in understanding and forecasting non-residential demand is that the non-residential properties
are, even within a segment, heterogeneous in their demand for water. The water demand varies significantly
due to different uses and different organisation sizes. An unfortunate implication is that it is difficult to find
explanatory variables that can be used as a basis of forecasting.
33 There is little value in undertaking any analysis of the trend models as the model forecasts are highly
dependent on subjective choices made to develop the model.
Page 26
Commercial in Confidence

developed. From the perspective of transparency and future analysis, it would have been
simpler if the forecast was based on some metric (e.g. the median) of the weather scenarios
(rather than median result from applying each scenario). However, I have no concerns with
regards to the accuracy of the approach used to incorporate these scenarios.
4.3.2 The recent adjustments
It appears clear that the 2015 Forecast has been under-forecasting actual demand and some
modification is required. I understand that the total number of non-residential properties is
similar to what was forecast and consequently the issue resides with the forecast average
demand per property.
The price adjustment
Sydney Water has assumed there was a larger price response than was incorporated in the
2015 Forecast. Based on this assumption Sydney Water removed the asymmetric price
adjustment, with the effect of doubling the impact of the reduction in price in effect (from
1.7% to around 3.5% of non-residential demand).
Without seeing additional information, it is difficult to assess the impact of price. I would
have expected that demand to be very inelastic in the short-run, particularly to downward
price movements. That is, I would not have expected firms to change water use significantly
in response to a downward shift in price. Over time I expect the effect would be larger and
more in line with the elasticity referred to in the literature.
The assumption regarding price need not matter. I understand that no further real price
changes are expected for the next pricing period and therefore the effect of this adjustment
is a one-off. If there are no further price changes, then, due to the final adjustment, the price
adjustment is of no real consequence. That is, if the price adjustment were not made, the
final adjustment would be larger to compensate and there would be no change in the total
non-residential demand forecast.
Adjustment for stronger population growth
A second reason for the stronger demand is that there has been stronger residential
population growth than forecast (which has not been accompanied by stronger growth in
non-residential properties). A consequence is that there are more people in work places who
are using water. Sydney Water suspects that average demand on some non-residential
properties (e.g. commercial properties) has increased due to higher population density.
In my opinion it seems reasonable to expect that demand per non-residential property would
be at least partly driven by residential population density and so some modification in this
regard is appropriate.
34 […] although trends in average demand were examined, it was concluded that for all segments/systems any
recent movement in average demand per property was insignificant. On this basis the forecast for average
demand per property was set to be equal to average recent demand.
35 For example, in each case slightly different results might be achieved by using a slightly different period for
selecting the average demand and property growth. However, the subjective choices made appear to be
reasonable, to have been given appropriate consideration and to be made without bias.
Page 27
Commercial in Confidence

Sydney Water’s adjustment involved increasing the 2015 Forecast by the ratio of the revised
population forecast to the population forecast that existed (the 2012 forecast) when the 2015
Forecast was made.
This adjustment has some significance. If the adjustment was not made there would be a
larger final adjustment; however, the final forecast would differ slightly because the final
adjustment is based on historic differences and the population adjustment increases over
time.
In my opinion, the population-adjustment assumption does not seem unreasonable.
However, it would be preferable that the assumption be further examined by examining how
average demand has changed by property category.
Final adjustment
The final adjustment involved inflating the total aggregate demand by an additional 1.1 GL
per year, estimated as the average difference between the forecast (including the above two
adjustments) and the ‘actuals’ for the prior 8 years.
I have two concerns with the final adjustment relating to the data used in adjustment
calculation and the weather variation in the hindcast period.
Calculation procedure for the final adjustment
The final adjustment was calculated as the average of the actuals less the adjusted non-
residential forecast. Unfortunately, the non-residential forecast used did not include the
necessary adjustment for WICA properties (see the last step in section 4.1.2), resulting in the
adjustment—and the final forecast—being 0.1 GL higher than if the error had not been
made. The error is small; It represents less that <0.1% of the non-residential forecast and
<0.02% of the overall forecast and is minor relative to the risk of weather variation.
There was also an issue with the ‘actuals’ used in the calculation, which I understand to have
been of no consequence. The ‘actuals’ used in the calculation were not adjusted for an
adjustment (called back-coding) of properties that were originally classified as ‘Other billed
consumption’ but were reclassified as ‘non-residential consumption.’ I understand this
adjustment was not made when calculating the ‘Other billed consumption’ amount (which
was based on a simple historical average) and consequently the issue did not affect the
overall forecast.
Weather variation
A challenge in assessing the accuracy of this adjustment is that there is variation in the model
errors. The 8-year period covers a period (2010-11 to 2011-12) when the model
overestimated demand and a period (2009-10 and 2013-14 to 2016-17) where model
underestimated demand.
As noted in information provided by Sydney Water, the errors tend to be correlated with
weather conditions. As shown in Box 7 below, the model:36
•
overestimates in years with relatively low temperatures and evaporation
36 Sydney Water found no clear relationship with average rainfall, which they thought was ‘probably due to
sensitivity to distribution over year.’ Source: Modifications to non-res model Presentation.
Page 28
Commercial in Confidence

•
underestimates in years with relatively high temperatures and evaporation.
This correlation between the errors and weather conditions may be a result of the use of the
6-monthly dummies in the regression models.
A consequence of this correlation is that the accuracy of the adjustment will likely depend on
how the weather variables in the hind-cast period (the prior 8 years) compares with the
expected weather in the forecast period. If the weather in hind-cast period is representative
of the forecast period, then the adjustment made from the hind-cast period will (from the
perspective of weather) be appropriate.
Box 7: Correlation of weather and forecast error
Figure 3: Weather and forecast error
The charts on the left show the residual
errors against the average key weather
variables for each of the 8 years in the
hind-cast period.
As highlighted in the figures there is a
correlation between the weather variables
and the residual error.
I understand the vertical line in each figure
is similar to the average weather in the
forecast scenarios.
As described in section 3.1.3, the forecast is based on a best-case drawn from forecasts
developed from 100 different synthetic weather scenarios. I understand that the average
weather from these scenarios is similar to the vertical lines in the figure in Box 737 and
therefore (all else being equal) the appropriate adjustment should be similar to what would
occur when the weather variables are similar to the vertical line. In such case, based on a
37 Based on discussions with Sydney Water staff.
Page 29
Commercial in Confidence
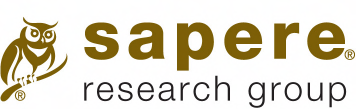
casual observation of the figures, it appears possible the forecast error—and consequently,
the appropriate adjustment—could be smaller than 1.1 GL.
4.3.3 Other billed consumption
Other billed consumption (‘Other’) includes water used on properties which have not been
allocated a property type. This amount was added to the non-residential forecast to create a
total ‘non-residential plus other’ forecast. I understand ‘Other’ was estimated to be 4 GL per
year based on a previous forecast. More recent data suggest that this is slightly high.38
4.3.4 Modelling results
The aggregate modelling results are summarised in Figure 4 below, which shows the actual
results, the unadjusted hindcast, adjusted hindcast and forecast for the ‘non-residential +
other billed consumption’ demand. As reflected in the figure, the forecast is for very little
change in the aggregate forecast.
Figure 4: Summary of non-residential forecast
Annual non-residential + other consumption (GL/ year)
130
125
120
115
110
105
Actuals
Hindcast
Hindcast adj
Forecast
38 The ‘Other’ category includes metered-usage for properties which due to a change in the associated property
number no longer have a property-type. A process of back-coding was undertaken to reclassify the historic
consumption. Once this procedure was undertaken, the average annual volume for the 8 years to 2016-17
for ‘Other’ was ~2.67 GL. However, the back-coding procedure also involved reallocating ~0.97 GL to the
non-residential demand which was not incorporated in making the final adjustment. Combined the historical
amount for ‘Other’ is around 3.63 GL per year. The back-coding also involved reallocation of some
historical consumption to the ‘residential’ category. However, the residential forecast procedure did not rely
on aggregate consumption and so is unaffected by this procedure.
Page 30
Commercial in Confidence
4.3.5 Conclusion
The non-residential demand forecast is effectively a modification of 2013 Forecast (and 2015
Forecast) with a revised approach to forecasting weather and some adjustments to account
for recent discrepancies.
At a high-level the ‘non-residential plus other’ forecast involves:
•
estimation of the average future demand by property segment
using models estimated in 2013 that include controls for price and weather
based on the assumption of no changes in price and the median result from future
weather scenarios developed by an independent research group at UNSW
•
multiplied by the forecast number of properties based on trends determined in 2013
•
with adjustments to address discrepancies between the actual and forecast.
In the absence of time and budget constraints, it would have been preferable to re-estimate
the models using recent data. However, I do not expect this would have resulted in a
materially different result given the adjustments made.
In my opinion, the proposed forecast is not unreasonable.
As is discussed above, I have identified two small issues. My primary concern is that the
underlying model under-estimates the effect of weather variation, which—given the unusual
weather conditions in the hind-cast period and the adjustments used to calculate the final
adjustment— could lead to the forecast being too high. In addition, there is was a minor
error in the calculation of the final adjustment relating to the treatment of WICA licences.
Page 31
Commercial in Confidence